How to Design a Data Warehouse Step-By-Step: A Comprehensive Guide
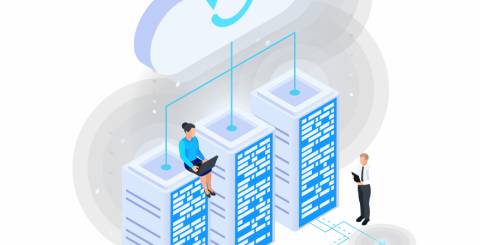
Designing a data warehouse is a strategic activity that builds the groundwork for strong data management and analytics capabilities within a business. In today's data-driven world, the systematic creation of a data warehouse is not only a technical requirement but also a critical step in harnessing the power of information for informed decision-making.
In this article, I will show you how to design a data warehouse that matches smoothly with your business objectives. You will learn the key steps and principles of data warehouse design, from conceptualization to implementation. We will also provide insights and best practices from various sources to help you create a data warehouse that will serve as the foundation for data-driven success.
Understanding Data Warehouse Design Methodologies:-
Before embarking on the journey of designing a data warehouse, it's essential to be familiar with the methodologies commonly employed in the industry. Two widely recognized approaches are the Inmon and Kimball methodologies.
- Inmon Methodology: Inmon's methodology focuses on the development of a centralized data repository known as the "Corporate Information Factory." This methodology focuses on combining data from multiple sources into a unified, comprehensive data warehouse. The data is then translated into a consistent, standardized format. While this strategy necessitates extensive planning, it guarantees data consistency and correctness.
- Kimball Methodology: The Kimball approach promotes dimensional modeling, which organizes data into "star schemas" or "snowflake schemas." This process is more iterative, enabling incremental development and speedier deployment. It stresses end-user accessibility and is ideal for enterprises with changing business needs.
Step-by-Step Approach to Data Warehouse Design:-
Now, let's break down the process of designing a data warehouse into a step-by-step guide:
Understand Business Goals: Begin by collaborating closely with stakeholders to define and understand the organization's business goals. Identify key performance indicators (KPIs) and determine the data needed to support strategic decision-making.
Identify Relevant Data Sources: Conduct a thorough analysis of existing data sources within the organization. This may include transactional databases, spreadsheets, flat files, and external data repositories. Evaluate the quality and relevance of each data source about the defined business goals.
Define Data Warehouse Architecture: Based on the chosen methodology (Inmon or Kimball), define the overall architecture of the data warehouse. Determine whether a centralized or distributed approach is most suitable for the organization's needs.
Plan ETL (Extract, Transform, Load) Process: Develop a detailed plan for the ETL process, which involves extracting data from source systems, transforming it into the desired format, and loading it into the data warehouse. Consider factors such as data cleansing, data validation, and transformation rules during this stage.
Create Data Models: Design the data models based on the chosen methodology. The Kimball approach, involves creating star schemas or snowflake schemas, while the Inmon approach focuses on normalized data structures. Pay attention to the relationships between dimensions and facts to ensure data integrity.
Implement Security Measures: Define and implement security measures to safeguard sensitive data. Establish role-based access controls and encryption protocols to protect data at various levels.
Optimize Performance: Fine-tune the data warehouse for optimal performance. This includes indexing, partitioning, and implementing caching mechanisms to enhance query performance and reduce response times.
Implement Data Governance: Establish data governance policies to ensure the accuracy, consistency, and reliability of data within the warehouse. Define data stewardship roles and responsibilities to maintain data quality over time.
User Training and Documentation: Provide training sessions for end-users and create comprehensive documentation to assist users in navigating and extracting valuable insights from the data warehouse.
Monitor and Maintain: Implement a robust monitoring system to track the performance of the data warehouse continually. Regularly update and maintain the system to accommodate evolving business requirements and technological advancements.
Final Words
Designing a data warehouse is a multifaceted process that requires careful planning, collaboration, and attention to detail. By understanding the organization's business goals, selecting an appropriate methodology, and following a step-by-step approach, you can build a data warehouse that serves as a strategic asset, empowering decision-makers with timely and accurate information. Remember, the key to success lies in adaptability, continuous improvement, and a commitment to meeting the dynamic needs of the organization.
Similar Articles
Unless you have been hiding in a cave somewhere, you would know and realize that the world is creating information at a stunning speed. While it is genuinely considered normal information that said data can now be turned into the groundwork of achievement for essentially any business in the present day and age.
Software development refers to the procedure of constituting and nourishing software applications. This provokes the utilization of many fundamentals and practices. Software development targets constitute structured, dependable, and beneficial software.
The integration of Internet of Things (IoT) technology into the construction and real estate sectors, which include buildings, infrastructure, homes, and businesses, is predicted to increase dramatically in the future. Despite this predicted expansion, the construction industry is behind other industries in terms of IoT adoption.
In this dynamic world of innovative and transformative technology, the use of Minimum Viable Product (MVP) has proven to be a winning strategy for success.
While monolithic applications may have waned in popularity during the era dominated by the cloud and microservices, interest is resurgent. Organizations, in considering their position on the application modularity spectrum, are now examining both the advantages and drawbacks of relying on microservices.
Data visualization is an indispensable tool that allows us to transform raw, and often unstructured data into insightful visuals, identify patterns, and communicate these insights to the wider audience and stakeholders.
For modern businesses to thrive, ensuring the effective management of inventory stands has become vitally important. Inventory management stands as a cornerstone of success. And the emergence of the Internet of Things (IoT) has introduced a new era of connectivity and efficiency across diverse industries.
Do you know what the following e-commerce companies have in common: Amazon, Walmart, eBay, and more? All of these e-commerce companies' apps make use of Java. Java is decidedly among the leading choices of programming language for e-commerce applications because it offers a world of benefits; for example, since Java code can be run on any platform with a Java Virtual Machine (JVM), users of e-commerce apps made with Java can access the said apps on a variety of devices.
Nikola Tesla in 1926, once described what is now called a mobile phone as a telephone that can fit into one's “vest pocket.” As otherworldly as that idea was then, nearly a century later, the reality is even more astounding.